Bayesian network integrated testing strategy (ITS-3) Summary of defined approach
This method as described by Jaworska et al [1] is designed to improve precision and accuracy for predicting and replacing the in vivo Local Lymph Node Assay (LLNA) assay. It makes a prediction for skin sensitisation potency calculated in the form of a probability distribution over 4 sensitisation classes (non-sensitiser, weak, moderate and strong sensitiser). Classification of a compound is based on its pEC3 value, which is the result of the LLNA assay or the result of Bayesian network (BN) prediction. The probability distribution is then transformed to a Bayes factor to remove prediction bias from the training set distribution and to give a quantitative measure to the level of uncertainty which can then be used in an objective manner to assign a confidence level to the predictions. Our implementation of ITS-3 is based on 207 chemicals for which physico-chemical data, in vitro, and in vivo data are available. We use Chemaxon tools to calculate water solubility at pH 7, logD at pH 7 and LogKow. These descriptors in addition to the TIMES in silico model prediction, and our proprietary protein binding estimate are used in combination with the information from the validated in vitro assays provided by the user to arrive at a prediction.
BN approach uses a knowledge-based network of connections among the nodes. Each node represents a single model variable and each connection between the two nodes represents how the variables influence each other and the final pEC3 value. A training set of compounds is employed to estimate the influences among the model variables. Subsequently, the in vitro data and physico-chemical properties of the testing set are assigned to the input nodes and the probability distribution over pEC3 values is computed based on the established connections/influences among the variables.
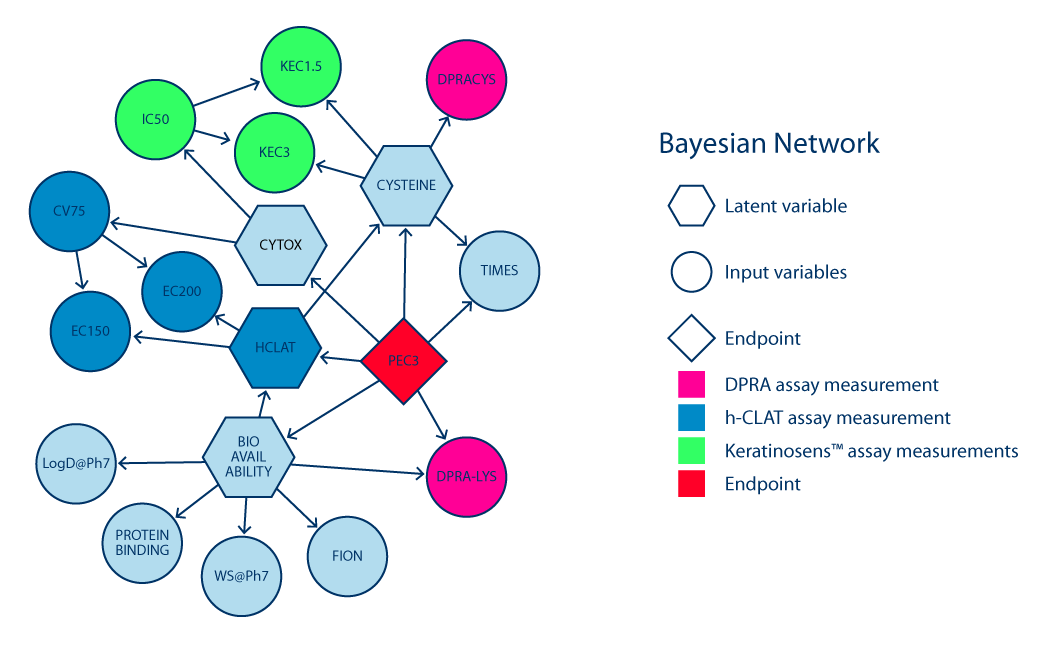
Table 1 shows the prediction accuracy for SaferSkin™ as compared to the results published by Jaworska et al. The statistics of the original network are based on the published confusion matrix.
Table 1 Calculated accuracy per pEC3 class for the original ITS-3 network in article as well as the reproduced Bayesian network.
Class | Jaworska et al | SaferSkin™ |
---|---|---|
C1 | 92% | 95% |
C2 | 82% | 77% |
C3 | 70% | 68% |
C4 | 72% | 79% |
overall | 79.6% | 79.6% |
Advantages of the Bayesian network approach include:
- It tolerates missing information.
- It conveys a probabilistic hypothesis of skin sensitisation based on accumulated evidence from data.
- It assesses the uncertainty in prediction given the input data.
- It suggests the experiments to maximize information gain and reduce uncertainty through the value of information (VoI) analysis.
Experimental values used in SaferSkin™
Input parameters | Provided by user | Calculated by application |
---|---|---|
SMILES | ||
Molecular descriptors | ||
TIMES prediction | ||
Michael acceptor | ||
Water solubility @ pH7 | ||
LogKow | ||
Protein binding | ||
logD @ pH7 | ||
Experimental values | ||
DPRA assay: DPRACys, DPRALys | ||
KeratinoSens™ assay: KEC1.5, KEC3, IC50 | ||
h-CLAT: EC150, EC200 and CV75 |
(1) J. S. Jaworska, A. Natsch, C. Ryan, J. Strickland, T. Ashikaga and M. Miyazawa;Archives Toxicol. 2015