SaferSkin™ web interface Tutorial
The SaferSkin™ Application provides Integrated Approaches to Testing and Assessment (IATA) that assesses skin sensitisation potency based on the following five approaches:
- ‘2 out of 3’ Voting: A majority voting model that uses three different types of in vitro and in chemico tests to predict whether a chemical is a skin sensitiser or not in the LLNA assay. This is one of the defined approaches proposed by the OECD TG 497 guideline1 . The required inputs for this model include: (i) Cys and Lys protein depletion capacity of a compound measured during the DPRA assay, (ii) the 1.5 and 3 – fold sensitisation gene expression capacity of a compound at a given concentration observed during the KeratinoSens™ assay, and (iii) CD54 and CD86 dendritic cell activation capacity of a compound measured during the h-CLAT assay.
- OECD ITS (Integrated Testing Strategy): A decision tree and scoring model that uses DPRA, h-CLAT in vitro assays and in silico tools (OECD QSAR TB or Derek Nexus) to predict GHS skin sensitisation potency class. This is one of the defined approaches proposed by the OECD TG 497 guideline1 .
- Multiple Regression: A simple and robust machine learning model based on multiple linear regression that uses inputs from kDPRA, KeratinoSens™ and compound’s vapour pressure to predict compound’s potency as the pEC3 value in the LLNA assay2 .
- Neural Network: A machine l Neural Network: A machine learning model that uses three different types of in vitro, DPRA, KeratinoSens™ and h-CLAT assays and in silico inputs (TIMES-SS and/or ToxTree) to predict skin sensitisation potency as the pEC3 value in the LLNA assay 3,4,5,6 .
- Bayesian Network: The model uses molecular descriptors for its classification of a compound into skin sensitiser or skin non-sensitiser, including the octanol/water partition coefficient (logKow), the octanol/water partition coefficient (logD), the water solubility, the protein binding capacity and if the compound is a Michael acceptor or not. To increase the prediction accuracy, the experimental values from DRPA, KeratinoSens™ and h-CLAT assays as well as the in silico QSAR TIMESSS result can be included.
Step 1: Submit your molecule
The following steps pertain to the Professional version:
- Enter the SMILES of the molecule into the designed field or use the option to draw the structure.
- If you have an SDF file, simply drag and drop it into the drawing panel. This action will load the 3D structure of the molecule.
- Alternatively, before trying your molecule, you can select a molecule from the provided list to explore the application.
- Press the "GO" button to initiate the process.
- The chemical structure of the molecule in question will be displayed under the "Prediction" panel.
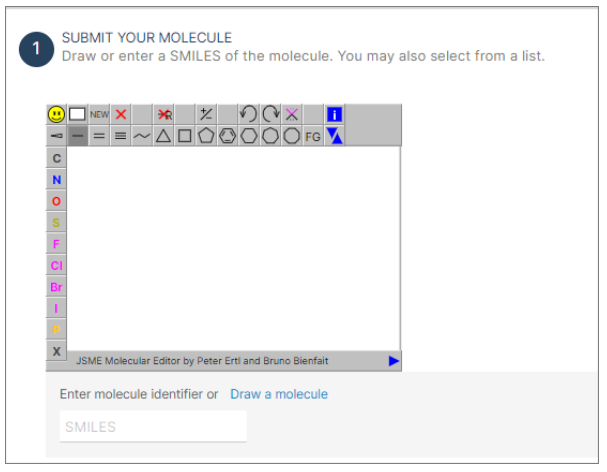
Step 2: Molecular Descriptors Generation
- After submitting the molecule, by pressing the Go button, the automatic generation of molecular descriptors will initiate.
- Allow a few minutes for the calculation of molecular descriptors to complete.
- The molecular descriptors will be displayed under the Bayesian Network section of the interface.
- If you have more accurate estimates or if there are missing pieces of information, you may modify the displayed values as needed.
- At this stage, you can use the molecular descriptors to predict the skin sensitisation potential of the molecule:
- Press the "Make a prediction" button to initiate the prediction process.
- Please be aware that the confidence level of this prediction is considered weak as only molecular descriptors are submitted.
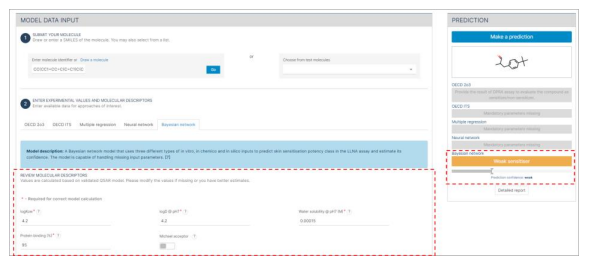
Step 3: Experimental Values Input
If you have experimental values available from the validated alternative assays, follow the steps below to input them. These assays include:
❖ DPRA (Direct Peptide Reactivity Assay)
❖ KeratinoSens™
❖ h-CLAT (Human Cell Line Activation Test)
- Input the experimental values of each assay in the appropriate field. - For detailed information about each assay and the expected input values: Click on the "?" buttons next to each assay entry field.
- When values are entered for a specific assay in one interface, these values will be automatically filled in the corresponding assay field of any other related interface.
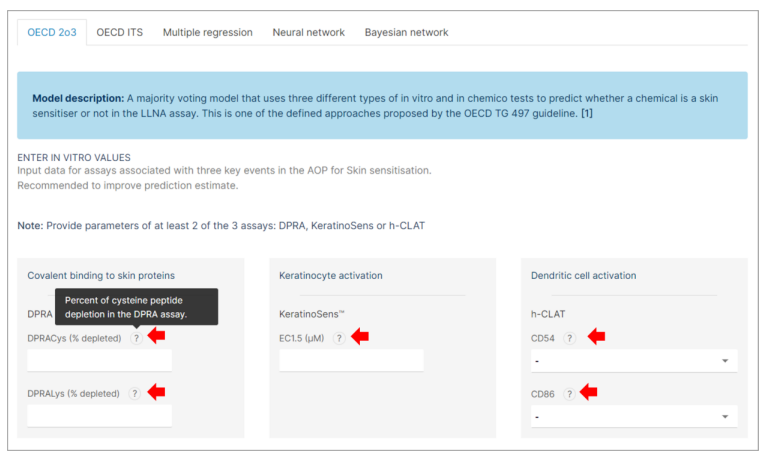
Step 4: Physico-Chemical Property Value Input
- Find the section of "Multiple regression" interface.
- Click on the field labeled as "Vapour Pressure". This field is mandatory and must be filled to proceed with the analysis. It is marked with an asterisk (*) to indicate its importance.
- Enter the numerical value of the vapour pressure as specified by the interface (Pa).
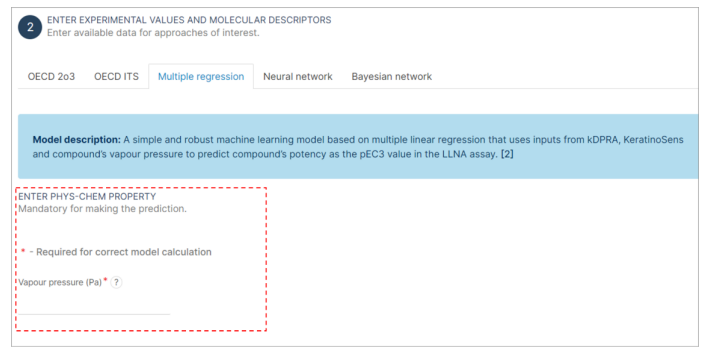
Step 5: in silico Data Input
- Choose from the following interfaces to input your data:
❖ OECD ITS (in silico input is mandatory)
❖ Neural Network
❖ Bayesian Network
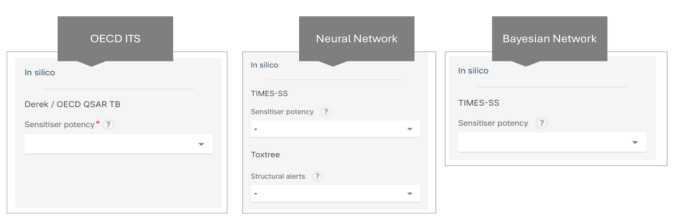
Step 6: Prediction
- Click on the blue "Make a prediction" button at the top.
- Check under all the sections for its prediction, labeled as "Not a sensitiser" (Green), "Weak sensitiser" (Light Orange), "Moderate Sensitiser" (Orange), "Strong Sensitiser" (Red).
- The label differs for OECD 2o3 and ITS. Please check the label in the following table:
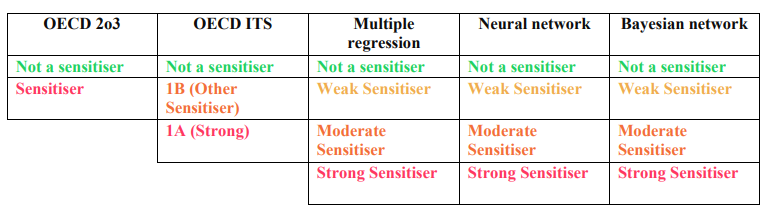
- Look at the "Prediction confidence" slider to understand the confidence level, which is marked as "weak", "substantial" or "strong".
- If the necessary values are not provided or are incomplete, it will not be possible to make predictions due to the missing mandatory section.
- Click on the "Detailed report" button at the bottom for an in-depth analysis.
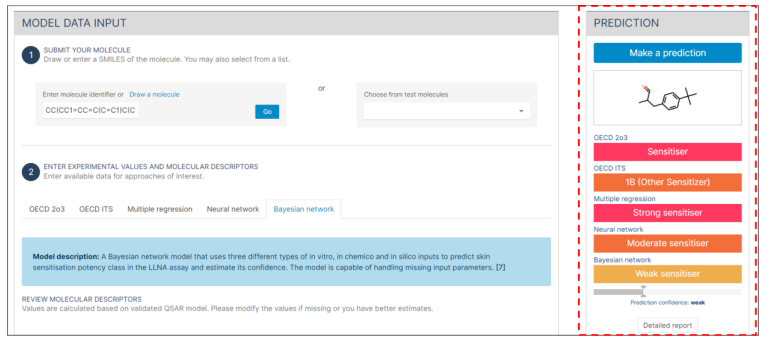
Step 7: Report
7.1. Report Analysis
A summary of report is presented at first which includes the binary classification (sensitizer/not a sensitiser) for OECD DA 2o3 and the three-tier GHS potency classification (1A, 1B and not a sensitiser) for ITS, as well as the four-tier potency classification (not a sensitizer, weak sensitiser, moderate sensitiser, or strong sensitiser) available for Multiple regression, Neural network, and Bayesian network approaches.
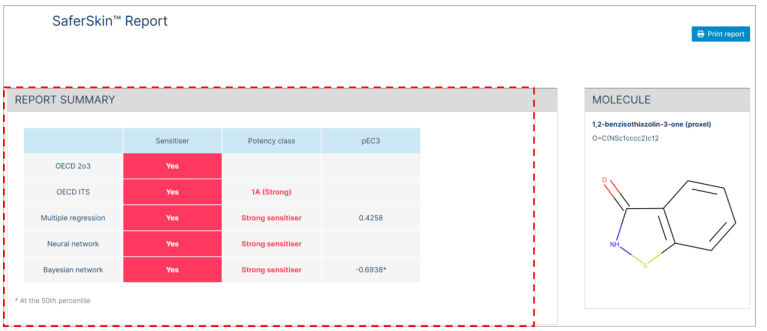
Following the summary report, a detailed report for each approach is presented. Each detailed report is composed of "Safety assessment guide" (e.g., sensitisation category, EC3, pEC3), "Input parameters" (experimental values, molecular descriptors, in silico prediction), and "Calculated values" section generated for the Bayesian network approaches.
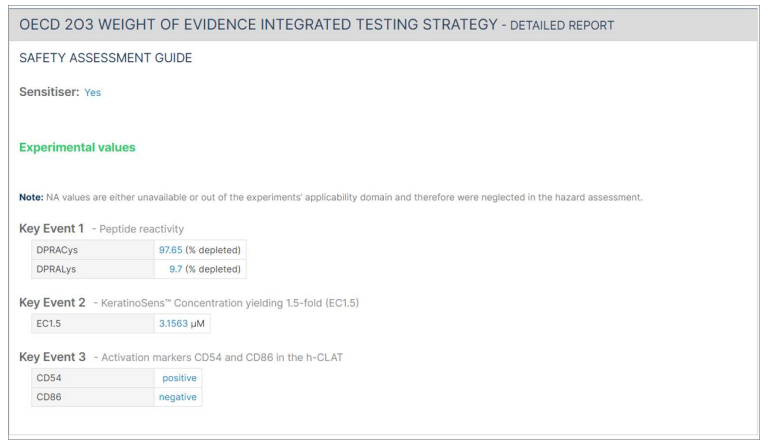
The OECD ITS detailed report also includes a display of the battery score.
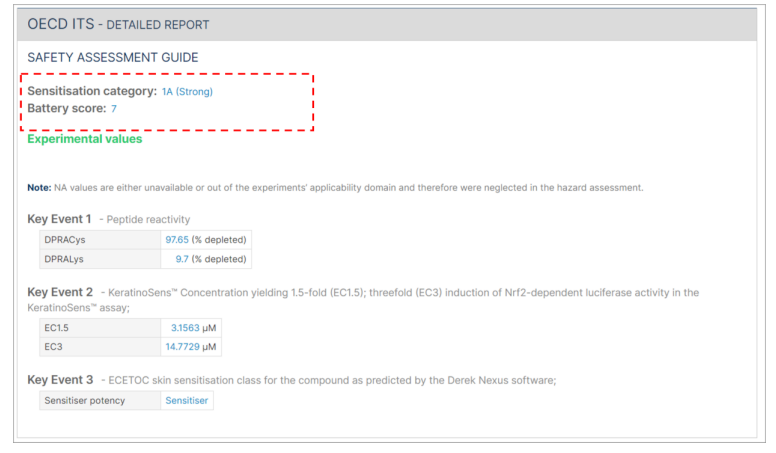
The Multiple regression detailed report includes the pEC3 result and the sensitisation category. The sensitisation category (1 = Not a sensitiser, 2 = weak sensitiser, 3 = moderate sensitiser, or 4 = strong sensitiser) is derived from the pEC3.
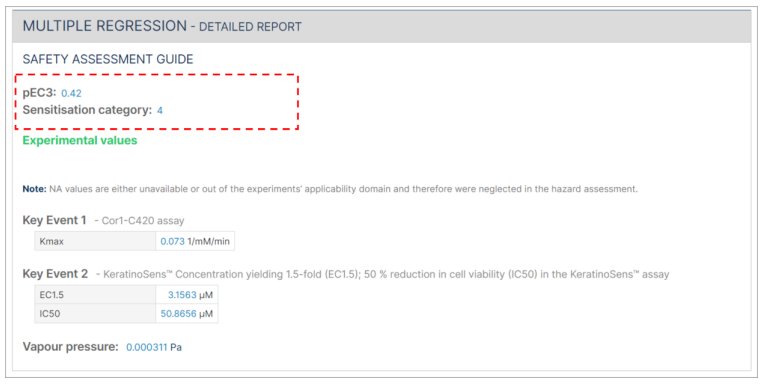
In cases where not all required information is submitted, the safety assessment will indicate "NA" instead of providing a pEC3 value.
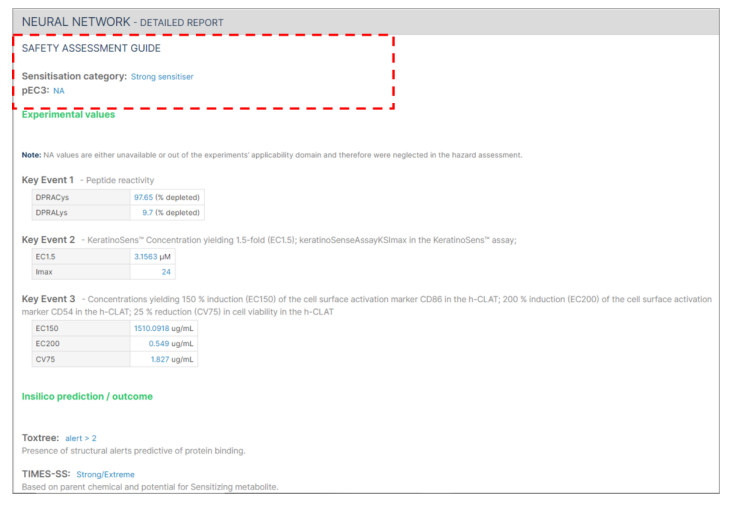
The "Safety Assessment Guide" for the Bayesian network detailed report includes the EC3 and pEC3 at all the percentiles (50th, 60th, 70th, 80th and 90th). A potency probability distribution based on exploring the 50th - 90th percentiles of the pEC3 for the molecule is generated.
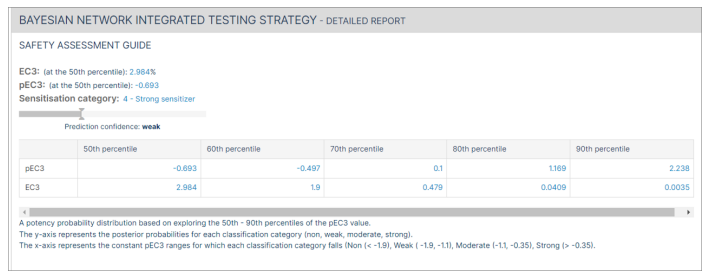
The "Calculated values" section is specific to the Bayesian network model, which includes:
- Prior Probabilities: The prior probabilities are always: Non= 0.2653, weak= 0.2653, moderate= 0.2721, Strong= 0.1973 (as calculated from Jaworska et al. 20157 )
- Posterior probabilities: The calculated posterior probabilities may change with each molecule prediction.
- Michael acceptor correction: In the instance where a compound is designated as a Michael acceptor, the posterior probabilities are adjusted to account for the anti-inflammatory properties of the compound.
- The Bayes factor is an indication of the strength of evidence for accepting the prediction. It quantifies the uncertainty to aid in decision-making.
- The compound is classified based on the category with the highest Bayes factor. The larger the Bayes factor, the stronger the evidence to support a prediction. <1 = Negative (evidence supports an alternative); 1–3 = Weak; 3–30 = Substantial; >30 = Strong.
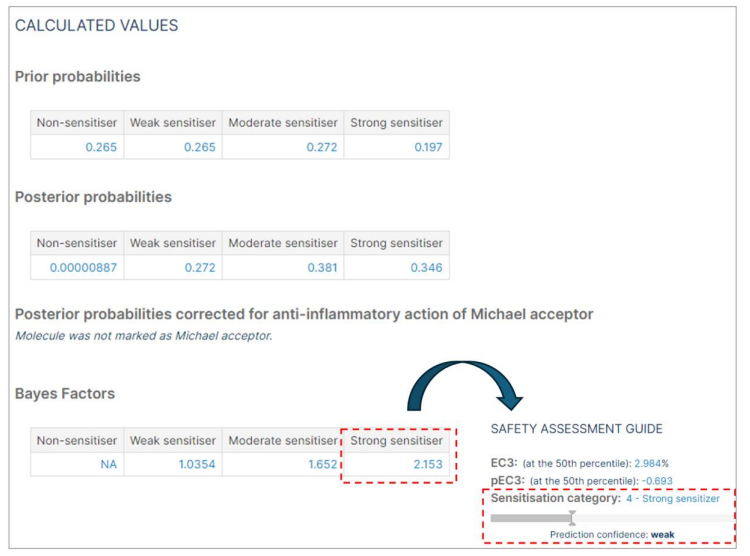
7.2. Printing/Saving Report
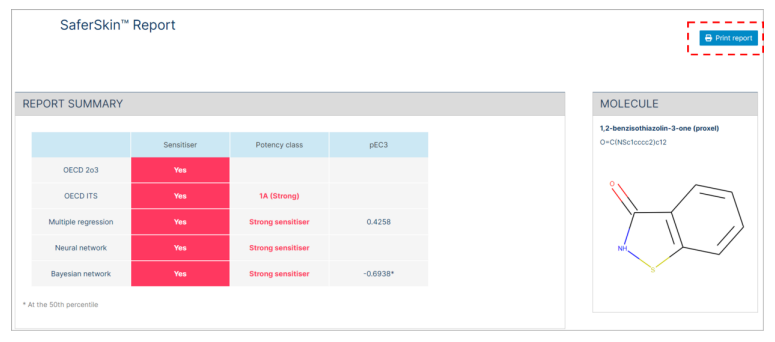
When you click the "Print Report" button, the result report will be generated and can be saved as a PDF or printed.
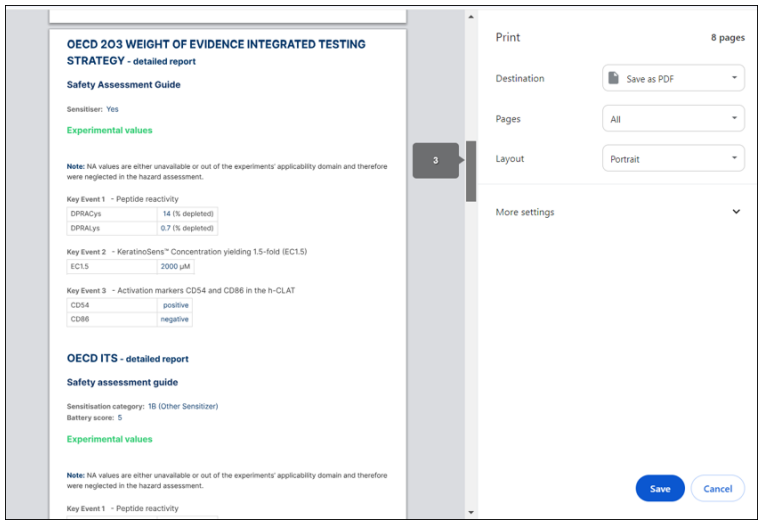
References:
(1) OECD Guideline No. 497: Defined Approachs on Skin Sensitisation https://www.oecd-ilibrary.org/environment/guideline-no-497-defined-approaches-on-skin-sensitisation_b92879a4-en
(2) Natsch, A., Emter, R., Gfeller, H., Haupt, T., & Ellis, G. (2015). Predicting skin sensitizer potency based on in vitro data from KeratinoSens and kinetic peptide binding: global versus domain-based assessment. Toxicological sciences : an official journal of the Society of Toxicology, 143(2), 319–332.
(3) Im, J. E., Lee, J. D., Kim, H. Y., Kim, H. R., Seo, D. W., & Kim, K. B. (2023). Prediction of skin sensitization using machine learning. Toxicology in vitro : an international journal published in association with BIBRA, 93, 105690.
(4) Hirota, M. et al. (2015). Evaluation of combinations of in vitro sensitization test descriptors for the artificial neural network-based risk assessment model of skin sensitization. Journal of applied toxicology : JAT, 35(11), 1333–1347.
(5) Kleinstreuer, N. C., et al. (2018). Non-animal methods to predict skin sensitization (II): an assessment of defined approaches . Critical reviews in toxicology, 48(5), 359–374.
(6) Hirota, M., Ashikaga, T., & Kouzuki, H. (2018). Development of an artificial neural network model for risk assessment of skin sensitization using human cell line activation test, direct peptide reactivity assay, KeratinoSens™ and in silico structure alert parameter. Journal of applied toxicology : JAT, 38(4), 514–526.